The financial services industry is, therefore, undergoing a revolutionary change, with the heart of it being Artificial Intelligence (AI). AI technologies have changed everything from personal finance management to risk assessment and fraud detection. Through the deployment of AI, financial establishments enhance efficiency in their operations, raise the level of customers’ experience, and make data-driven intelligent decisions. Be it processing loan approvals or personalized investment advice, AI does all that’s needed in this industry.
This lengthy blog post shall outline the many facets of AI within the realms of finance, ranging from credit scoring and lending to financial forecasting, fraud detection, and reporting. In this long blog post, we also take a deep look into the challenges facing AI within the realms of finance, as well as its potential for the future. Lastly, we use cases, examples, and case studies for AI’s applications within finance.
The Role of AI in Financial Industry and its Impact:
Artificial intelligence refers to the simulation of human intelligence processes by machines, especially computer systems. In finance, AI employs aspects such as ML, NLP, predictive analytics, and robotic process automation in improving an organization’s processes, decision-making outcomes, and even customer experience customization. AI is not a single technology but rather a network of tools and techniques that are interconnected to help automate and optimize financial services.
How AI Innovations is Shaping Finance Industry?
Machine Learning in Predictive Analytics
Machine learning is one of the AI branches in which algorithms learn from historical data in order to establish the trends and outcomes. It is heavily applied in the financial industry, in terms of credit scoring, algorithmic trading, fraud detection, and financial forecasting. The models that are made with machine learning are pretty good at picking patterns in enormous sets of data, and so it provides the people of finance with better decision-making processes.
Predictive analytics is a subset under machine learning and is commonly employed for the forecasting of future financial outputs using past data. Predictive analytics can be used in the banks to forecast the interest rates, loan defaults, and trends in the stock market. Armed with such information, financial analysts and institutions make strategies, change their portfolios, and reduce risks.
Natural Language Processing (NLP)
NLP enables machines to understand and process human language. In finance, automation of reports, customer support, and sentiment analysis are commonly used. For example, through AI-powered chatbots, a customer can be assisted by getting data pertaining to him or her, answering queries of customers, even managing their accounts, therefore relieving human staff.
NLP can also be highly informative in financial reporting AI bots wherein financial reports can be prepared automatically and disseminated without time waste and mistakes. Also, on deep unstructured data like posts or tweets on social media, news, and research reports, NLP can extract valuable information that helps in investment decisions and risk assessments.
Robotic Process Automation (RPA)
RPA is a software that uses software robots to automate rules-based, repetitive tasks. Within finance, RPA will increasingly be used in back-office operations, which involve data entry, account reconciliations, transaction processing, and regulatory compliance checks, so financial institutions can enhance the efficiency of their operations by reducing errors and costs.
For example, an RPA bot can be programmed to automate checking customer identity documents for validation or update huge numbers of financial statements in accounts payable software with repeated data entry. Such process automations could free up some of the tasks from the human employees so they can be allocated to more value-added activities like strategic decision-making or interacting with customers.
Use Cases of AI:
AI Application in Management of Personal Finance
AI is empowering individuals and is giving them the power in terms of controlling their personal health. AI-powered personal finance management tools provide users with an easy experience in regard to budgeting, savings, investments, and eventually financial planning for the future.
These tools use machine learning and provide tailored advice and automate everything except most aspects of one’s financial management.
AI Personal Finance Management APIs
AI-powered personal finance management APIs revolutionize the way people connect to financial data. The APIs allow developers to include AI features to be used in their applications, offering end-users a broad range of financial services such as the following:
- Expense Tracking: AI can track the patterns of expenditure of a person and gives insight into what can be reduced or conserved.
- Suggesting Investments: AI checks with the risk tolerance and other financial goals of a customer to provide the best and most suitable investment strategy so that people could easily increase their wealth.
- Automated Budgeting: AI will categorize all spending based on historical behavior, suggesting a budget plan and assisting users to hit their financial targets.
For instance, apps such as Mint and YNAB have AI that automates the execution of various personal finance tasks, tracks expenses, and provides much-needed financial guidance. With AI-driven insights and recommendations, these tools now offer financial planning to more masses.
Free AI Budgeting Tools
Now, owing to the emergence of plenty of free AI tools, this can be achieved with easy monitoring of one’s account without necessarily having to use expensive financial advisors. These categories of free AI-based budgeting tools can aid individuals in tracking income, and users even set savings goals while allowing savings suggestions based on how people should spend their money based on machine learning algorithms which study individual spending habits over time.
Among the commonly used free AI budgeting tools are Mint, a machine-learning engine to track transactions and create budgets and offer personalized financial advice by directly linking with the users’ bank accounts and credit cards in giving real-time updates on their status.
YNAB is an AI application that will guide users in proactive budgeting, hence the proper use of money and a plan for spending. Most parts of budgeting are automated, which gives users the control over their finances.
These tools help people get to know more about their financial situation and make wise decisions without necessarily needing professional knowledge or assistance.
AI in Credit Scoring and Lending
AI brings significant changes into the ways through which loans get assessed and eventually granted. Traditional models on credit scoring will never get to address all of these factors considered in giving someone access to lending. A better look at several data points will make use of an AI when a better assessment could be based upon sources in non-traditional data.
AI Powered Fast Loans
Lending AI-driven platforms are transforming loan processing. Through the use of algorithms and alternative data, machines learn and can ascertain, on the spot, the lending risk, hence making allowances easier for lenders on behalf of borrowers for fast loans.
AI-based credit apps, such as Upstart and LendUp, score its borrowers based on education, job history, and even social behavior, rather than on the traditional credit scores, allowing faster loan approvals and opening up considerable credit access for those with a limited or no credit history.
AI Lending Companies
AI lending companies are using machine learning to improve the loan approval process, enhance the risk assessment process, and optimize lending decisions. Platforms like Zest AI, Kiva, and Funding Circle apply AI models that assess more data points from the borrower than credit scores do for assessing their risk.
For example, Zest AI creates an AI-driven underwriting model of credit based on alternative sources of data; these are educational background, history of transactions, and social behavior. Such a view helps the AI lender to provide better customized loans to people having unusual or uncommon credit history, thereby accessing funds easier for them in cases where the traditional system would reject their funding application.
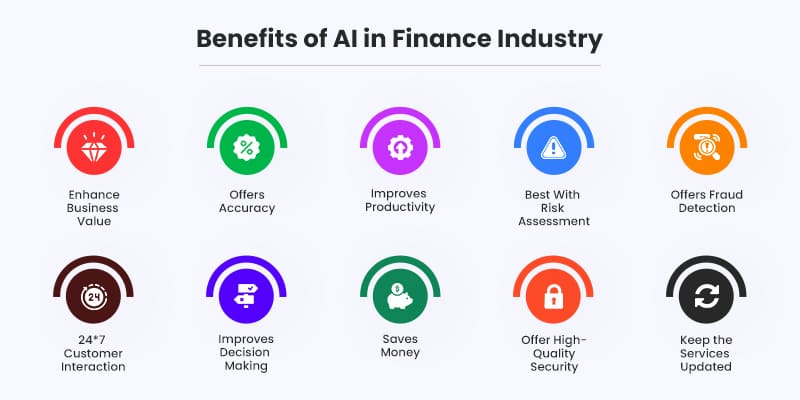
Source: Matellio
Customized Offers of Loans
AI enable lenders to create the most bespoke loan offers on the basis of a client’s financial profile and increase their chances of getting accepted for credit and successful repayment. AI-based lending companies use machine learning to help them determine the best loan terms and interest rates and repayment schedule for a given borrower.
AI in Financial Risk Management and Fraud Prevention
Mitigation of financial risks represents an important aspect of finance operating. AI is changing financial and banking institutions’ nature related to risk management approaches to using highly sophisticated forecasting predictive tools and identifying various malicious activities, such as fraudulent actions.
AI for the Detection of Fraud
AI revolutionizes fraud detection with systems capable of analyzing real-time transactions and flags possibly fraudulent activities. Machine learning models can identify fraud patterns of which even a human analyst may not be aware.
For example, PayPal utilize AI-powered fraud detection systems to review transactions as they happen. It can potentially identify abnormal activities, like big withdrawals or transactions from places the customer is not familiar with, and immediately block such activity for further review.
Another emerging fraud prevention AI tool is behavioral biometrics. This measures how a user interacts with their device, such as typing speed, mouse movements, and tapping on the screen, and flags up any irregularities that may be signatures of fraudulent behavior.
AI in Financial Risk Assessment
Financial institutions are also being assisted to evaluate and manage their risks. Machine learning models, through an analysis of very large data sets, can predict potential risks in market volatility, fluctuation prices, or credit defaults and come up with very useful insights to enable the institution make even better decisions.
For example, financial modeling AI gives banks and financial institutions the ability to forecast an entire range of market scenarios, thereby allowing them to identify risk factors and mitigate these risks. Such simulations are crucial so that financial portfolios remain resilient to changes in the market.
AI in Financial Reporting and Compliance
Financial reporting and compliance are part and parcel of the finance sector. AI is also important in streamlining institutions as they cut down on the burden of manual reporting, and still get them to comply with the regulatory standards.
AI for Financial Reporting
Other significant automation roles of AI are in automating the preparation of the financial reports; for instance, balance sheet, income statement, and regulatory filling. Automation on these improves accuracy, diminishes human errors, and thus gets the work done within due time in financial institutions.
The most significant advantages of financial reporting AI bots is that it can take a huge volume of data and produce reports much more quickly than human employees. The closing process in finances is much faster, and firms can focus on making strategic decisions.
AI in Regulatory Compliance
The complexity and changing nature of financial regulation are challenging. AI is enabling compliance tasks, including risk assessment, audits, and reporting to regulatory bodies, to be automated. By use of AI, the institutions will be able to follow the changes in the landscape and ensure they comply with all these laws, such as Sarbanes-Oxley, MiFID II, and GDPR.
The use of machine learning in AI-powered regulatory technology (RegTech) enables real-time monitoring and assessment of compliance. It also warns institutions of potential violations, which might save them costly fines. It saves both time and resources since manual effort in staying compliant is reduced.
Conclusion: The Future of AI in Finance
Artificial intelligence is no longer some future technology in finance-it is already a robust tool that changes the manner financial institutions function. AI can bring significant improvements from lending and risk management, through fraud prevention to customer services for the financial services sector-very efficient, secure, and accessible.
Future generations of AI technologies only enhance the prospect of them in finance. As machine learning, natural language processing, and predictive analytics continue to evolve and mature, financial organizations will unlock so many more avenues for innovation and efficiency. But these very improvements raise quite vital questions in areas of data privacy, security, and ethics about decision-making by AI, with which the industry will grapple as it moves on.
Ultimately, the future of AI in finance lies in continuous growth. Only those early adopters who will be best placed to offer smarter, more personal focused services and competitively outstrip others in an ever more data-driven world will succeed.
If you are financial business looking to get started with AI solutions exclusively, Ajackus is here to help you. You can get in touch with us to know more about our offerings. Let’s speak!
Start a Project with Ajackus